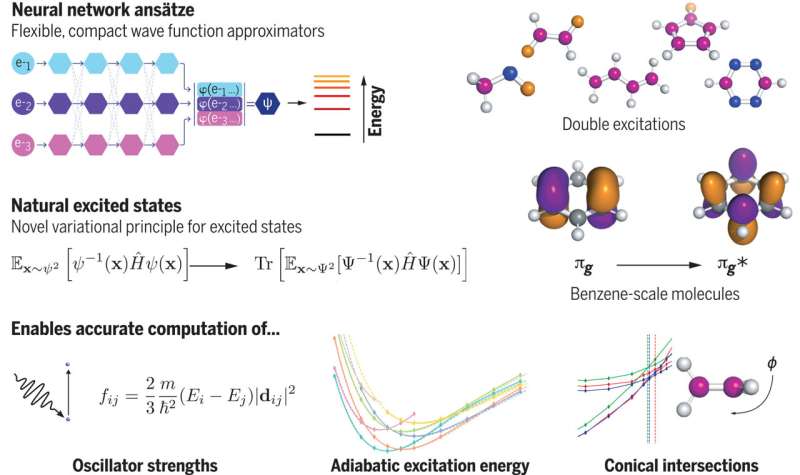
New research employing neural networks, a type of brain-inspired AI, presents a promising approach to modeling molecular states. This technique could advance the ability to solve complex equations in molecular systems, paving the way for practical applications such as prototyping new materials and chemical syntheses through computer simulations before actual lab experiments.
The study, led by scientists from Imperial College London and Google DeepMind, was published in the journal Science.
Modeling Excited States
The research focuses on how molecules transition between excited states. When molecules or materials receive substantial energy, like from light or high temperatures, their electrons move to temporary configurations known as excited states. This energy absorption and release creates a unique "fingerprint" for each molecule or material, influencing technologies such as solar panels, LEDs, semiconductors, and photocatalysts, as well as biological processes like photosynthesis and vision.
Modeling these transitions is challenging because excited electrons are quantum particles, meaning their positions are probabilistic rather than fixed.
Dr. David Pfau, a lead researcher from Google DeepMind and the Department of Physics at Imperial, explained, "Representing the state of a quantum system is highly complex. We need to account for every possible electron configuration, and the space of these configurations is vast—representing it as a grid with 100 points per dimension would exceed the number of atoms in the universe. This is where deep neural networks can make a difference."
Advancements with FermiNet
The team developed a new mathematical model and applied it using a neural network called FermiNet (Fermionic Neural Network). This represents the first instance where deep learning has been used to compute the energy of atoms and molecules from fundamental principles with high accuracy.
Testing their approach on the carbon dimer, a small but intricate molecule, the researchers achieved a mean absolute error (MAE) of 4 meV (millielectronvolt), which is five times closer to experimental results compared to previous gold standard methods with an MAE of 20 meV.
Dr. Pfau noted, "We applied our method to some of the most complex systems in computational chemistry, including scenarios where two electrons are excited simultaneously, and found our results within about 0.1 eV of the most precise calculations to date."
The team has made their work open source, inviting the research community to further develop these methods and explore new interactions between matter and light.